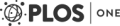
Abstract:
Recent research has focused on the monitoring of global{scale online data for improved detection of
epidemics, mood patterns, movements in the stock market political revolutions, box-oce revenues,
consumer behaviour and many other important phenomena. However, privacy considerations and the
sheer scale of data available online are quickly making global monitoring infeasible, and existing methods
do not take full advantage of local network structure to identify key nodes for monitoring. Here, we
develop a model of the contagious spread of information in a global-scale, publicly-articulated social
network and show that a simple method can yield not just early detection, but advance warning of
contagious outbreaks. In this method, we randomly choose a small fraction of nodes in the network and
then we randomly choose a friend of each node to include in a group for local monitoring. Using six
months of data from most of the full Twittersphere, we show that this friend group is more central in
the network and it helps us to detect viral outbreaks of the use of novel hashtags about 7 days earlier
than we could with an equal-sized randomly chosen group. Moreover, the method actually works better
than expected due to network structure alone because highly central actors are both more active and
exhibit increased diversity in the information they transmit to others. These results suggest that local
monitoring is not just more efficient, but also more effective, and it may be applied to monitor contagious
processes in global-scale networks.